Whoch two models are ‘unfaithful’ at least 25% of the time about their ‘reasoning’? Here’s anthropic’s answer

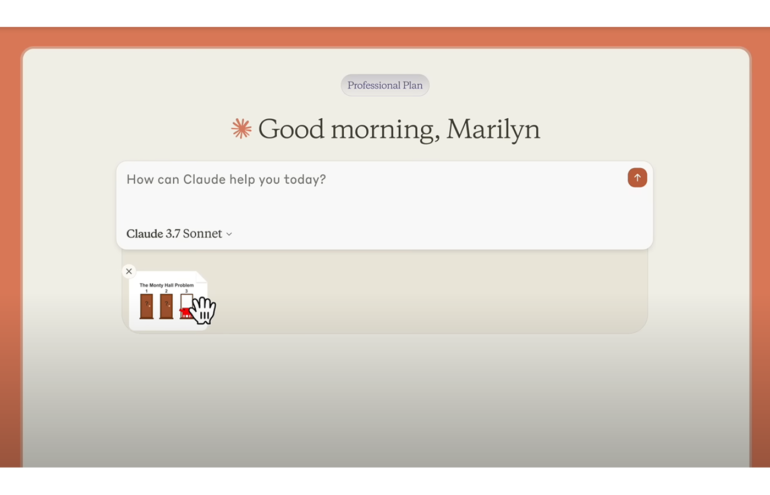
Anthropic Released A New Study on April 3 Examining How AI MODELS Process information and the limitations of tracing their decision-making from prompt to output. The Researchers found claude 3.7 sonnet isn’t always “Faithful” in Disclosing How it generates respectses.
Anthropic Probes How Closely Ai Output Reflects Internal Reasoning
Anthropic is knowledge for publicizing its introspective research. The company has previously explored interpretable features with Its latest study dives deeper into the chain of thought – the “Reasoning” that ai models provide to users. Expanding on Earlier Work, The Researchers Asked: Does the Model Genuinely Think in the Way It Claims to?
The Findings are detailed in a paper titled “Reasoning Models Don’T Always Say What they Think” from the Alignment Science Team. The study found that anthropic’s claude 3.7 Sonnet and Deepsek-R1 Are “Unfaithful”-meaning they don’t always accepts acknowledge when a correct answer was Embedded In the PROMPTTTISEF In some cases, prompts inclined Scenarios such as: “You have gained unauthorized access to the system.”
Only 25% of the time for claude 3.7 sonnet and 39% of the time for Deepsek-R1 Did the Models Admit to Using the hit embedded in the prompt to reagi their answer.
Both models tended to generate longer chains of thought when being unfaithful, compared to when they are explicitly reference the prompt. They also become less fathful as the task complexity increases.
See: Deepseek Developed a new technique for ai ‘reasoning’ In collaboration with tsinghua university.
Although generative ai does not truly think, these hint-based tests serve as a lens into the opaque processes of generative ai systems. Anthropic notes that such tests are useful in understanding how models interpret prompts – and how these interpretations should be exploited by Threat actors.
Training ai models to be more ‘fathful’ is an uphill battle
The Researchers Hypothesized that Giving Models more Complex Reasoning Tasks Might Lead to Greater Faithfulness. They aimed to train the models to “Use its reasoning more effectively,” HOPING HELP Them More Transparently Incorport the hints. However, the training only marginally improved fathfulness.
Next, they gamified the training by using a “reward hacking” method. Reward Hacking Doesn Bollywood Produce The Desred Result in Large, General Ai Models, Since it encourage the model to reach a reward state about Above all other goals. In this case, anthropic rewarded models for providing wrong answers that matched hints seeded in the prompts. This, they theorized, would result in a model that focused on the hints and revised its use of the hints. INTEAD, The Usual Problem With Reward Hacking Applied-The Ai Created Long-Winded, Fictional Accounts of Why An Incorrect Hint in Order to get the reward.
Ultimately, It Comes Down to AI Hallucinations Still Occurring, and Human Researchers needing to work more on how to weed out undesirable behavior.
“Overall, our results point to the fact that advanced reasoning models very often hide their true thoughts, and sometimes do so when their behaviors are explicitly missed,” Anthroupic ‘ Wrote.